The Balancing Act Between AI Ethics and Insurance Innovation
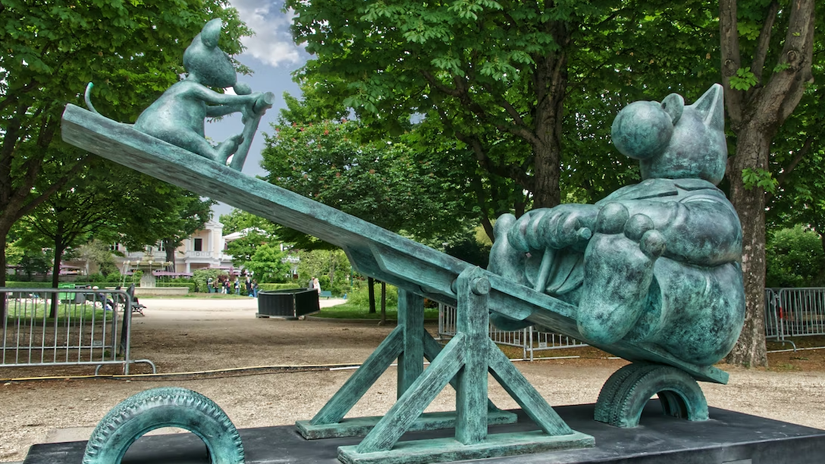
Machines thinking like humans are not like Agent Smith, in the Matrix trilogy. The sentient bad guy assumes the form of a white male in a black suit, frameless dark glasses and an earpiece. He belongs to a class of AI programs known as “Agents” who are tasked with protecting the Matrix and are superhumanly strong with contempt for humanity and think they need to be erased like a virus. That sounds exciting and terrifying but in truth, AI in everyday life is much more commonplace. It could be as simple as unlocking your phone with your face. There is a common factor though between Agent Smith and present-day AI programs - Is AI governed by any ethics and are there systemic AI biases that can creep in that could discriminate against people? And even more distressing, could AI come to conclusions without the need to explain the process because after all, they learn from experience?
As AI becomes the fuel that is powering innovation, particularly in highly regulated industries such as insurance, AI ethics can no longer be glossed over.
Why AI bias can have far-reaching implications for stakeholder trust
Insurance companies are using AI and machine learning to automate parts of the claim handling process and to improve customer service. AI is also displaying its power to revolutionize the way we buy auto insurance because of telematics and smartphones. Newer technologies such as those deployed in usage-based insurance bring up the inevitable discussions on AI bias and data privacy.
AI ethics is relatively nascent compared to the evolution of artificial intelligence. We all know that artificial intelligence is the simulation of human intelligence in machines by training them to think by feeding data into computer algorithms. When humans develop new technologies it normally starts simple and as it gets more advanced that is when questions of ethical usage start arising. Let’s put this in perspective with two examples. The first goes back to a time before AI and the second shows how bias can be unconscious when building AI algorithms.
Until 2011, women were 47% more likely to be injured in a car crash. Further, fetal death was the highest cause of maternal trauma from a car crash. Digging deeper into this, it was found that manufacturers did not take into account the impact that the seat belt design would have on pregnant women. Even more surprising was that since auto manufacturers were using male bodies as test dummies for test crashes, the design effect on women was never studied. There was no malice in this oversight, just that new technology was being tested by the people who built it and they were males.
The next example is more recent from the financial sector where a large regional organization used a fraud detection algorithm to identify potential cases of fraudulent transactions. The algorithm was trained on a set of data to understand what a fraudulent transaction would look like compared to a normal one. Since it was dealing with big data, the fact that it contained a larger sampling of fraudulent applicants over 45 years old flew under the radar. This oversampling continued over months without being detected and the model began to consider that older folk were more likely to commit fraud. Only when regulators started asking questions because customers were raising complaints was the AI bias detected,
AI in everyday applications also shows such bias. For instance, voice recognition software has difficulty interpreting female voices. Again the reason is that R&D teams testing this software are normally dominated by men.
These examples underline the fact that by the time businesses come to a realization of ethical risk the effects are never small. Of course, AI will scale up operational efficiencies, risk mitigation and fraud detection. The other side of the coin is that if there are no checks and balances then AI ethical risk can have a massive negative impact on brand perception, a legal and financial impact, and also a loss of customer trust that is often painstakingly built through the years.
Also Read: AI for Insurance - Everything You Need to Know
The checks and balances involved in responsible AI
It is important to make the distinction that data models used to train machine learning algorithms are not biased, rather it is the training data that can render them biased. So how does insurance move forward with innovative technologies when customers are looking for proof of fairness? The solution will probably involve both external factors such as progressive regulatory standards, and internally through a corporate cross-functional lifecycle process for AI model governance.
Regulatory compliance
The AI technology wave is still too new for lawmakers to fully understand how this technology will impact citizens. It is still hard to know how this technology will be fully utilized and what are the possible repercussions. Apart from some existing data privacy laws, legislators globally are taking a wait-and-watch stance as this data from Cognilitica indicates.
When it comes to America, there are significant AI regulations in the pipeline. The Algorithmic Accountability Act drafted in 2019 did not pass the US senate. Now though, the White House is proposing an AI Bill of Rights to make AI systems more accountable and give consumers the right to transparency. As we wait for the outcomes on that, it is important to note that while the federal government has still to legislate on this, many states in the US have enacted AI ethical laws.
-
The California Automated Decisions Systems Accountability Act (Feb 2020) mandates that businesses in the state establish responsible AI processes to continually test for biases.
-
Baltimore and New York City have passed local bills that prohibit the use of AI algorithms in making decisions that could be discriminatory.
-
Alabama, Colorado, Illinois and Vermont have passed bills to create a task force to evaluate the use of AI in their states.
The Federal Trade Commission (FTC) with decades of experience protecting consumers' rights has also enforced 3 laws that affect developers and usage of AI. For instance, section 5 of the FTC Act prohibits deceptive practices such as racially biased algorithms. For insurance companies, the Fair Credit Reporting Act (FRCA) which is the third law, comes into play when an algorithm is used to deny people insurance or other benefits.
Finally, the National Association of Insurance Commissioners (NAIC) released a framework in 2020 for the ethical use of AI. Of the 5 tenets, the one on transparency states that stakeholders, including regulators and consumers, should have a way to inquire about, review, and seek recourse for AI-driven insurance decisions.
Also Read: New Policy Management Software: The Guide to Getting IT Right
Internal AI model governance
For the most part, insurance has been relatively transparent when it came to consumers and businesses buying insurance. If you live in a flood zone, for example, the relationship between risk and premiums is easy to understand. However, as big data and artificial intelligence take the burden off the shoulders of underwriters and claim adjudicators, more attention must go into providing greater visibility into decisions. This is what NAIC also recommends. Multiple frameworks are already being developed by the insurance sector in the US. They are specifically centered around how data is extracted and stored and how it is utilized to interact with customers.
The frameworks are also recognizing that AI because of its technical nature tends to silo data scientists and engineers from other stakeholders within the organization. Risk and compliance specialists usually come in at the end to evaluate a system that they had little say in, to begin with. To bring greater transparency will require insurance companies to not just focus on reliable data models but also to make core documentation accessible to everyone involved in the project. All project stakeholders must understand the outcomes and be convinced that the model developed is the best way to solve the problem. Creating this transparency will require putting in place objective governance that includes not only the decisions that the models make in deployment but also the human processes that surround the conceptualization, development and deployment.
However, even with the best intentions, humans are at the center of development and errors can still occur even with the best cross-functional governance in place. Business owners and other stakeholders should have technical dashboards that can evaluate system performance and know what course correction was made to identify any bias and rectify it. What comes across is that transparency is vital for all players involved in implementing new technologies, the customer being a vital part of it. Balancing commercial interests, and protecting proprietary technology while at the same time maintaining customer trust, appears a conundrum but one that needs to be solved.
Topics: A.I. in Insurance


