Brain vs Bot: Does RPA in Insurance Need Intelligent Automation?
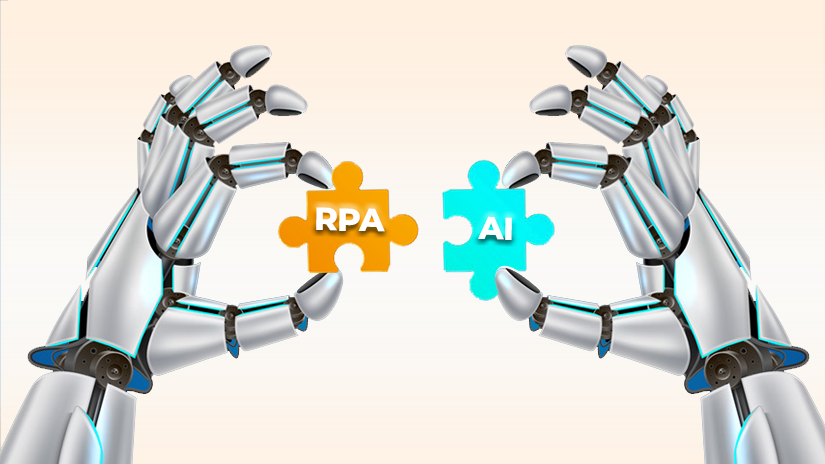
It is a well-recognized fact that the insurance workforce spends an average of 15% of their time on repetitive tasks. While these are pretty important tasks, such as manual underwriting or customer database updates, the point is that they are not exactly bringing in new customers or even helping personalize the customer experience, neither are they saving costs. Yet, they must be done, so just get on with it, right? Wrong, it might need to be done but why have humans do them if there is a better alternative like Robotic Process Automation.
Financial sector case studies (McKinsey) have shown a 200% growth in ROI in the first year of RPA deployment. This then is the reason why RPA has already got a wide adoption in the insurance industry to automate repeatable tasks in back-office operations. However, an RPA bot cannot ‘think’ and that is why it is more of a short-term solution. Insurance carriers looking to move to a digital transformation path need to use RPA coupled with intelligent process automation.
Why RPA can only be a starting point
Although RPA is used in almost all industries, the biggest adopters are in the banking sector, insurance carriers, and utility companies (Gartner). The reason is clear, these industries have a large number of well-defined processes that make them highly suitable for robotic process automation. To understand how insurance carriers are using RPA, we need to first understand how RPA works because that is where its limitations also become visible.
RPA is rule-based software that has no intelligence built into it. RPA technology predominantly uses UI (user interface) to create specific low-code scripts to automate routine tasks - tasks, that at most, have limited variations so that a finite set of rules will define what must be done. Visualize a bottling industry where assembly line machines work faster than humans and without a break - doing the same work day after day. That is exactly the same when it comes to RPA and business processes in insurance workflows. Take for instance claims processing, it requires companies to collect data from a large number of sources. It is exhausting but it is based on well-defined structural inputs and that is why programming languages can be written to automate data collection.
The challenge though is that RPA technology is too rigid, after all, it is based on a finite set of rules. Introducing RPA to replicate human tasks requires companies to keep adding new bots to cover new areas in which the previous technology was not programmed to handle. Further, processes change over time, RPA bots cannot pick up on these changes and have to be reconfigured for the particular change. That’s why while RPA is incredibly useful it is only part of the solution and not the full solution.
RPA needs to be augmented with cognitive intelligence to train the bots to learn from experience and evolve. Tech-driven startups like Lemonade and Metromile are making traditional insurance carriers realize that digital innovation requires machine learning and artificial intelligence to be woven into more and more workflows.
Hand-picked for you: Can Automated Underwriting Impact Quality of Your Customer Base?
Adding brains to the bots
Traditional RPA automation is applied to repetitive tasks but when AI is combined with RPA (RPA is considered a subset of artificial intelligence) then the process can even identify exceptions and deal with them without any external input. It can analyze large volumes of data, both external and internal, and can pull out insights that can be translated into specific actions.
I am sure you are getting the picture but let’s look at how it works in an automated claims processing workflow. Typically, each claim would take several days as data must be gathered from different sources, for instance, driver’s license, police reports, and vehicle damage pictures in case of an auto claim. Intelligent process automation begins from the point of claims intake, assessment, and final settlement of the claim. When the system receives the first notice of loss, the RPA bot will extract all the information from the request sent in and enter details into the claims system. This process has already been working for years but RPA working along with intelligent optical character recognition (OCR) software will automatically read information from unstructured documents like emails or even phone transcripts or paper documents. Now enters a cognitive bot that will check the form to see if there are any missing details. If there is none, and if it is below a previously set threshold amount, it is automatically approved and sent to the payments department. However, if there is missing information and the AI bot is meeting it for the first time, it sends it to a human agent and ‘watches and learns’ how the agent resolves it. The next time it comes across the same exception, it knows what to do. This 'learning' is what sets apart the new process from the older versions.
Almost 20-30% of automated processes require exception handling
This is where RPA works best when it has intelligent automation on its side. Robotic Process automation workflows are always linear. However, business processes rarely have a linear progression and AI coupled with RPA provides the ability to take different paths, each having a successful outcome. AI/ML algorithms perfect the exception handling mechanism over time to be able to work with the least amount of human supervision.
Automated claims process, reduces manual work by 80%, cuts down the time needed for processing the claim by 50% and reduces the cost of a claims journey by 30%.
Also of interest: Big Fish Alternatives in P&C Insurance Core Systems
The evolution of RPA
Initially, RPA bots were unattended as the rules were defined and they were programmed to complete a specific task. Today, this has changed with more attended bots at work. This is because intelligent process automation is enabling them to work as personal assistants to human agents. These cognitive bots are being used to collect relevant data in near-instant time and provide it to humans. For instance, in a customer call, all information related to the customer’s request can be presented in a matter of seconds. The same goes for underwriting where more personalized plans can be provided when all data is quickly collated.
By 2030, manual underwriting will no longer exist for most personal products across life and P&C insurance - McKinsey
AI-RPA pairing is also being integrated into chatbots. Simplesolve’s own interactive bot, Tara, has been built to support intelligent underwriting. She also assists agents in upselling additional coverages and limits based on data in the insurance application.
The biggest benefit for traditional insurers is that RPA creates an automation layer above the existing legacy systems. AI is then an extra decision layer added over this to automate the BPM process. While modernizing systems for a full digital transformation is important in the long run, intelligent automation in insurance can still be enabled with existing systems to optimize workforce processes for better efficiency.
Topics: Intelligent Automation


