Data Security and Privacy Risks Associated With AI and How to Overcome Them
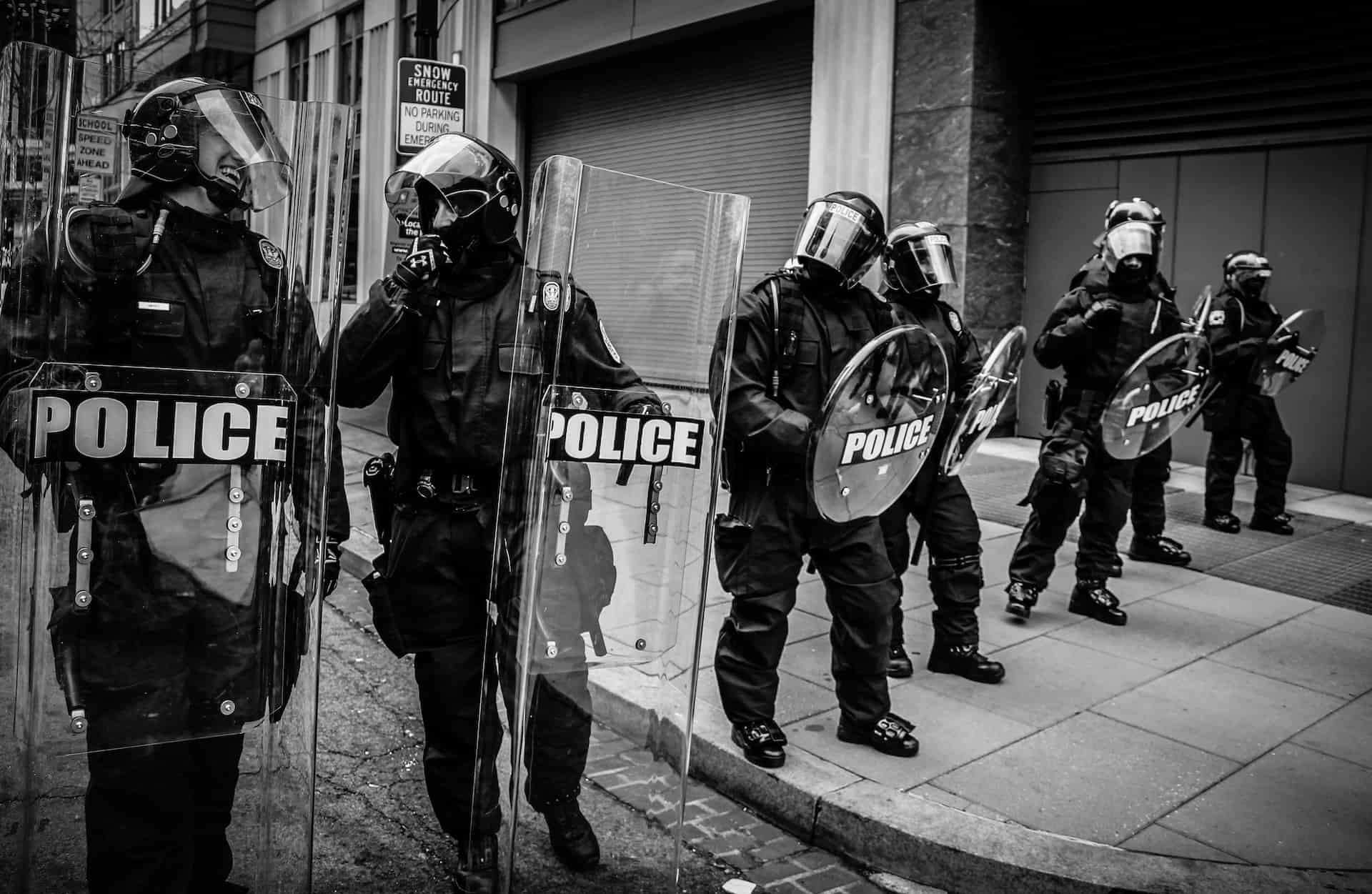
AI adoption in insurance signifies a paradigm shift in how the industry operates. In December 2022, the National Association of Insurance Commissioners (NAIC) published a report that says 88% of surveyed PPA (Private Passenger Auto) insurers use or plan to use AI/ML. Claims, marketing, and fraud detection are key areas of focus, while some are also using AI/ML for underwriting and loss prevention.
As American insurance companies increasingly harness artificial intelligence (AI) for insights and operational efficiency, data usage has grown into a more prominent and pressing concern. The transformative powers of AI solutions for insurance come with an evolving set of data security and privacy challenges. These risks of AI integration are not just because insurance has always been an attractive magnet for cybercriminals, there is also the additional complication of possible biases in the AI training models.
The importance of differential privacy techniques in AI data privacy
The vast scale of data collected and processed by AI makes it increasingly susceptible to data breaches and privacy violations. This necessitates stringent safeguards to maintain consumer trust through data confidentiality.
In designing AI products and algorithms, separating user data through anonymization and aggregation is a fundamental strategy for businesses leveraging user data to train their AI models.
AI systems thrive on data, and many online services and products insurers provide rely on personal data to fuel their AI algorithms. Recognizing the potential risks of AI data security lays the right groundwork for acquiring, managing, and utilizing data. These improvements encompass the algorithms and the broader data management infrastructure.
Recent research highlights the importance of differential privacy techniques in data handling, which adds an extra layer of privacy protection when utilizing sensitive user data. Additionally, ongoing studies emphasize the significance of robust consent mechanisms and transparency in data collection and processing practices, aligning closely with the evolving landscape of privacy regulations and user expectations.
Differential privacy techniques in data handling can be explained with two popular AI applications in insurance:
Example 1 for differential privacy: Claims Processing
Consider an insurance company using AI to streamline claims processing. Traditionally, this would involve examining individual claimants' data to assess risk and determine payouts. However, in the era of AI data privacy concerns, insurers want to protect policyholders' sensitive information now more than ever because some of the data may be outside the organization.
With differential privacy, the insurer can automatically add statistical noise to the claims data before processing it. For instance, when assessing the frequency of specific types of claims, the AI system might add random variations to the numbers, making it nearly impossible to identify individual claimants. This ensures that personal data remains confidential, while the AI still gains valuable insights into claims trends and risk assessment.
Example 2: Data Sharing for Risk Assessment
Insurance companies often collaborate and share data for a more comprehensive understanding of risk. Differential privacy can be applied in these scenarios to share aggregated insights while protecting the privacy of individual policyholders.
For instance, several insurers might exchange data about car accidents in a specific region to assess overall risk. Using differential privacy, they aggregate the data in a way that prevents the identification of individual accidents or policyholders. This collaboration helps insurers collectively assess risk without compromising individual privacy.
In traditional data-sharing practices efforts were made to aggregate and anonymize data, but the potential for privacy breaches still existed. The shift to using differential privacy represents a more intentional and mathematically rigorous approach to privacy preservation. It acknowledges and addresses the limitations of previous methods, providing a more robust framework to ensure that even in the collaborative sharing of data, the privacy of individual accidents or policyholders is more effectively safeguarded.
Privacy by Design as a factor to mitigate risks associated with AI
Privacy by Design (PbD) embodies a proactive strategy for safeguarding against possible risks of AI in data privacy. It emphasizes the seamless integration of privacy considerations into every phase of product, service, and system design and development. This approach ensures that privacy isn't just a feature but a fundamental principle from inception.
In the context of insurance AI solutions, Privacy by Design Principles has 7 core factors:
1. Proactive Not Reactive: Privacy measures should not be implemented as an afterthought or in response to breaches or regulatory mandates. Instead, they should be an integral part of the AI solution's design from the initial stages.
2. Privacy as the Default Setting: AI Privacy concerns should be the default mode of operation. Insurance AI systems should be configured to ensure the highest level of privacy, by default. Any deviation from this default should be a conscious choice made by the user.
3. User-Centric: Privacy by Design places the interests of policyholders and users at the forefront. Their preferences and concerns are taken into account in the design and operation of AI solutions.
4. Full Functionality: Privacy measures should not interfere with the primary functions of the AI system. Insurance AI solutions should be designed to deliver the required services and insights while still safeguarding data privacy.
End-to-End Security: Implement robust security measures throughout the data lifecycle, from data collection and transmission to storage and disposal. This ensures that data is secure at all stages.
6. Visibility and Transparency: Users and policyholders should have transparency into how their data is used and processed. They should be able to understand the data practices of the AI system.
7. Respect for User Privacy: AI systems should respect the privacy and confidentiality of data as a fundamental principle. Data should not be used for purposes beyond what was explicitly agreed upon.
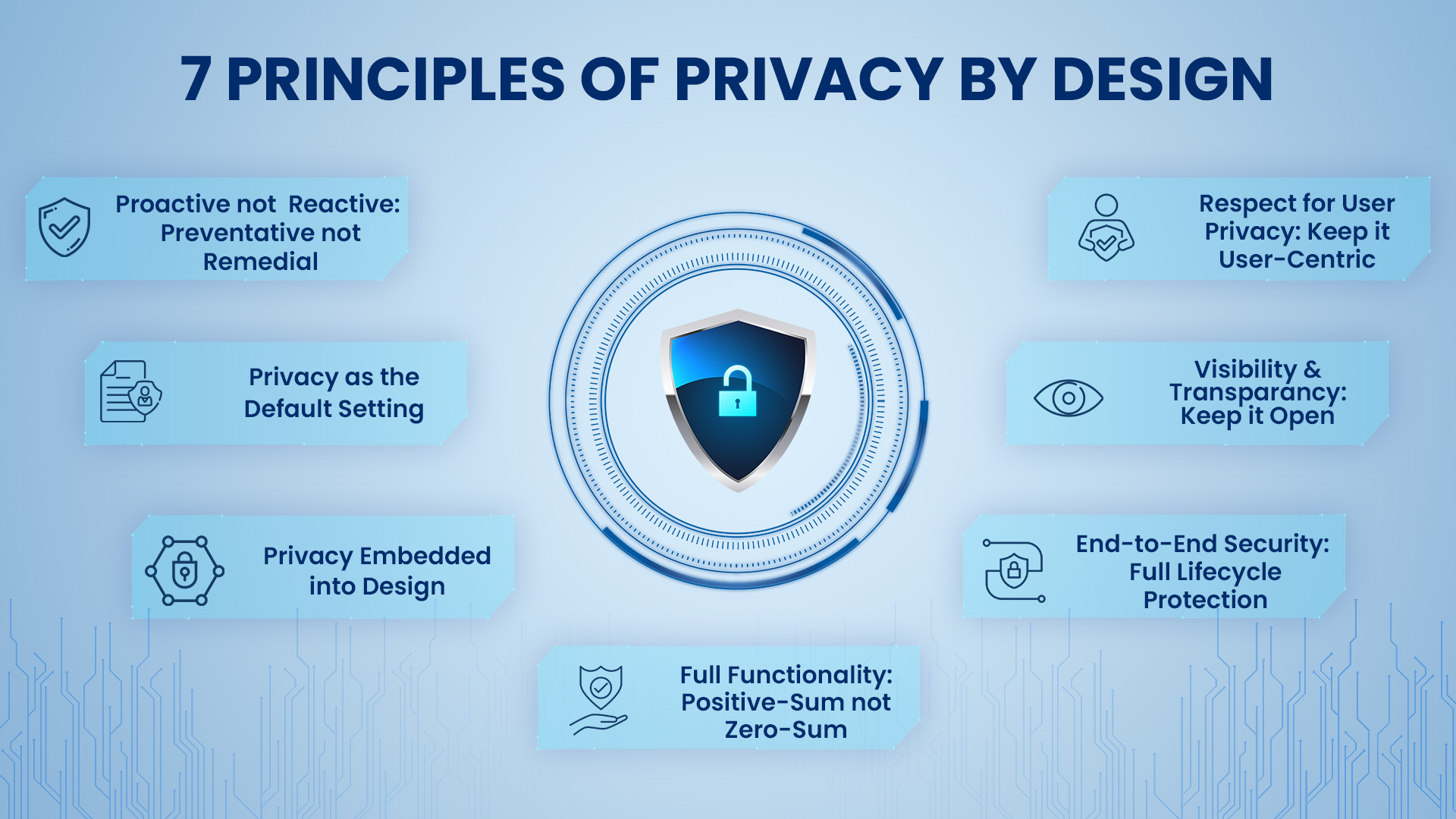
A "zero-sum" scenario is when one person's gain equals another's loss. In contrast, a "positive-sum" situation is a win-win, where all benefit without losses. It promotes cooperation and mutual gain.
Privacy measures should consider the entire data ecosystem, including third-party interactions, partners, and data-sharing agreements. Data Minimization should also be a criteria i.e. collect and store only the data that is necessary for the insurance processes. Minimizing data reduces the potential impact of a data breach and helps ensure that only the most essential information is handled
By implementing Privacy by Design in insurance AI solutions, insurers can build trust with policyholders, ensure compliance with privacy regulations, and proactively address potential privacy risks associated with AI.
Avoiding bias and discrimination in AI Models
AI technology introduces another significant challenge in the form of potential bias and discrimination. The fairness of AI systems hinges on the quality of the data they learn from; if the data carries bias, the AI system can perpetuate it. Such bias can result in discriminatory judgments impacting individuals based on criteria like race, gender, or economic status.
To avoid these pitfalls, it's essential to start with diverse and representative data for training AI models, ensuring a fair foundation. Conducting fairness audits can help identify disparities, allowing for model adjustments. Transparent and explainable AI models aid in understanding and addressing bias, while regular monitoring and retraining are key to keeping AI systems fair.
Implementing bias mitigation techniques, third-party audits, and feedback loops can further enhance fairness, as can setting ethical guidelines within the organization.
In the United States, ten states have incorporated AI regulations within broader consumer privacy laws that were enacted or will become effective in 2023. Furthermore, several more states have introduced bills with similar proposals. For instance, California's Insurance Code Section 1861.02 prohibits the use of certain factors in insurance pricing, aiming to prevent unfair discrimination.
AI technology deployed in high-risk environments like insurance necessitates a dual approach to governance. Human-centric governance ensures that human expertise, judgment, and ethical considerations remain integral in decision-making. It complements technology-driven governance, which encompasses safety measures embedded within AI systems. Together, these approaches strike a balance, leveraging the capabilities of AI while maintaining human oversight and ethical standards in critical contexts.
Schedule a Demo with us to understand how SimpleINSPIRE insurance platform integrates AI while safeguarding data privacy.
Topics: A.I. in Insurance


